How CPG and Retail Businesses Can Adopt Practical Data-Driven Marketing Strategies

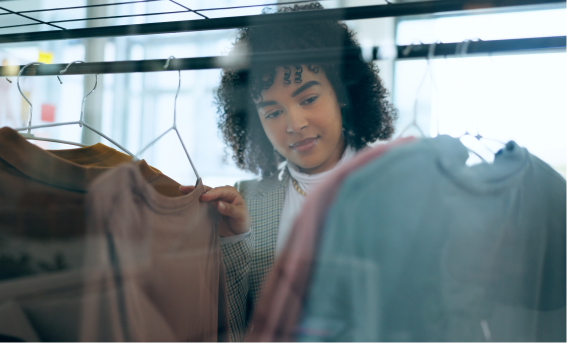
Leading an efficient, data-driven marketing organization in today’s business landscape can be daunting – the environment is one of complex marketing technology (MarTech), massive quantities of customer data, lightning-paced digital innovation, and emerging methods of machine learning and AI. Even large corporations face challenges orchestrating, measuring, and optimizing digital experiences and marketing campaigns to fuel successful go-to-market strategies.
In my nearly 20 years as a Marketing, Strategy, Insights, and Analytics expert working for and with CPG brands and Retailers ranging from startups to multi-billion dollar Fortune 100 corporations, I’ve seen approaches that span a wide spectrum, from companies that operate primarily on founder intuition to those relying on teams of 50+ of data scientists and analysts to inform marketing decisions. While companies tend to become more data-driven as they grow, it is not and should not be purely about company size or maturity. An analytic orientation is also a function of leader mindset, organizational culture and – perhaps counterintuitively – a real willingness to be creative, scrappy, and even imperfect when it comes to data-driven decision making.
There are many ways to begin to nimbly apply more data-driven approaches to marketing decisions even in small ways which, applying the 80/20 rule and a “don’t let perfect be the enemy of good” mentality, can yield big impact.
This article highlights five data-driven marketing lessons and provides specific examples from my experience implementing Marketing initiatives at various CPG and Retail companies:
- Build test & learn muscle, starting small
- Anticipate data needs up front, from the business perspective
- Start simple with personalization
- Crawl, walk, run with customer segmentation
- Find acceptable alternatives to gold standard measurement
Build test & learn muscle, starting small
Email is typically an organization’s highest-ROI marketing channel, and it is also the easiest one in which to build organizational muscle around experimentation. Via built-in features of most email service providers (ESPs), marketers can easily A/B test subject lines, pre-headers, content variations, promotional structures, copy, deployment timing, cadence, etc.
While many companies do test sporadically, they often fail to close the feedback loop by consolidating and systematically analyzing results across a series of tests, identifying patterns in results, generating and disseminating insights, and optimizing strategy based on the insights. The business and customer upside of improving email open rates, click-through rates and conversion can be easily quantified, which can in turn help the team justify a continued investment in a test and learn approach.
At a previous company where I worked, we implemented a rigorous email testing program and spent the time to analyze results, quantify dollar sales impact, share learnings, and codify our testing methodology for the organization. As a result, we influenced the culture to be more thoughtful about testing and learning for the purpose of delivering stronger results, which in turn helped justify investments in tools, capabilities, and partnerships to support testing of various promotional structures, campaign creative, website features, and more.
Anticipate data needs up front, from the business perspective
With scaling businesses, it is almost universally true that customer data is scattered, disparate, and siloed. Companies try to clean up and centralize the disparate data sources, aiming to get to a “360 degree view of the customer.” At the right stage of maturity, many companies now invest in customer data platforms (CDPs). Like the horror stories of failed ERP and CRM implementations of prior decades, it is not uncommon to hear stories of disastrous CDP implementations, leading to low adoption and ROI.
A lot of this has to do with a lack of upfront strategic consideration of the business needs and uses around consumer data – who are the business consumers of the data? How will they use it? Literally, how do they want to chart, map and visualize it? What business questions do they want to answer? It is critical to begin thinking about this in extreme detail long before any technical plans are solidified.
For example, a recent collaboration with an omni-channel apparel retailer involved analyzing customer data in a newly implemented CDP. The initiative had opened doors to uncovering valuable insights into customer segments and their purchasing habits, replacing previous decisions based solely on intuition. However, the product data feeding into the CDP was all at the bottom level of the product hierarchy, at the SKU level (e.g., Mens Dark Blue Moriah Style Sweater Extra Large UPC123456), with no mapping back to the full product hierarchy (e.g., Menswear items; Sweaters Category; Size Extra Large items). Additionally, there was no systematic rhyme or reason to the naming conventions. So, while we could start understanding who was buying each of thousands of individual SKUs, without significant manual work in Excel, we could not roll-up the data at a higher level to understand who was buying menswear or sweaters overall.
The team should have started out the CDP design exercise by outlining the key business questions and hypotheses, asking questions like, “Can we better understand who is buying our products?” Or, “Is it true our customers are women purchasing gifts?” Had they incorporated those questions into the upfront design, they would have recognized the need to refine product names and establish a structured product hierarchy to integrate into the CDP.
For another client, a lack of data governance and planning for a CDP implementation meant that dramatically too much data was sent over without considering business use cases, leading to 400% data overages versus contracted levels, resulting in huge unanticipated costs. In addition to unanticipated costs, there were too many customer attributes contained in the system, making it highly unwieldy for users, driving low adoption.
Start simple with personalization
While data scientists can create highly complex recommendation and personalization engines, even simple approaches can drive significant sales impact for companies without the resources of an Amazon or Netflix. Off-the-shelf recommendation engines can work for many sites, especially for organizations with relatively simple assortments. Even when developing custom personalization tools, starting simple and incrementally adding complexity can be the most effective approach.
For example, I worked with a major food & beverage retailer to better personalize the sorting of its digital coupon gallery to drive incremental coupon engagement, purchases, and trips. In order to move quickly, we started with a very simple algorithm based on business logic (not data science), surfacing coupons that customers had previously redeemed at the very top, followed by coupons in categories they engaged with next, knowing that in food & beverage, customers tend to stick to items and categories they’ve already bought.
While we started with intuitive business logic-based rules, we subsequently moved on to layer in more sophisticated, data-science and ML-driven approaches. In the end though, we found that the original, business logic-based approach was actually 90% as effective as the significantly more complex data science & ML approach. So, adding significant complexity and costs only gave us marginal upside.
Crawl, walk, run with customer segmentation
Another area that companies tend to become overwhelmed by – and therefore avoid entirely – is customer segmentation. New approaches to segmentation, especially in the DTC space, are based on behavioral data, and they can be highly complex.
On the other hand, RFM segmentation (based on the terms “Recency,” “Frequency,” and “Monetary Value”) is a relatively simple but powerful segmentation that can be done for any organization that has a database of customer-level data. The approach divides customers into quintiles along recency (how recently they purchased), frequency (how often they purchase), and spend (how much they’ve spent), and then creates segments based on that. It can be a relatively simple and effective way to begin to identify actionable segments, such as “Valuable but Lapsing” customers whom you want to save, “New and Promising Customers” whom you want to nurture, and “Top Loyalists” whom you want to keep happy.
Similarly, calculating Customer Lifetime Value correctly can be extremely valuable yet daunting, because it is a forward-looking, predictive metric. Academics and professionals have written books and earned their PhDs on the topic. Doing LTV accurately is not easy, so most organizations do not do it at all. While it is admittedly imperfect, I still see some value in taking a baby-step in the right direction, which is calculating LTV-to-date, which calculates backward-looking spend among customers (excluding new customers). It has limitations that are important to understand, but it is a place to start to understand differences in tenured customers and begin to identify differences between higher and lower value cohorts.
Find acceptable alternatives to gold standard measurement
Experimental design is the best way to demonstrate causality, which can enable you to definitively show that a change made to a website, or a new feature added to an app, had a clear impact on customer behavior and sales. However, it isn’t always possible to design a clean Test vs. Control experiment, and a data scientist isn’t always on hand to measure the results.
When I worked with a client to launch a major new feature on their digital app, for the sake of managing complexity, we needed to take a “flip the switch” approach and launch the new feature to users all at once rather than hold out a control group. To measure impact, we alternatively did a Difference in Differences analysis, which looked at whether the growth trend changed after the launch vs. before. While this is directional rather than conclusive of causality, results were highly encouraging. Due to technical improvements, we were later able to go back and actually execute a Test vs. Control design to validate results, and they showed that the Difference in Difference analysis was remarkably accurate. Similarly, when launching a loyalty program, the challenge of measurement always comes into play. We often need to come up with scrappy, directional, and creative ways to measure results, and it helps to come up with multiple measurement approaches in order to triangulate results.
In the end, becoming more analytical and data-driven in retail and consumer businesses is a cultural shift that comes from the top and requires internal champions and, sometimes, external support. It can be a stepping-stone process requiring incremental changes in mindset, technology and capabilities. But ultimately, it will yield results for your business and your customers.
Meet the Author
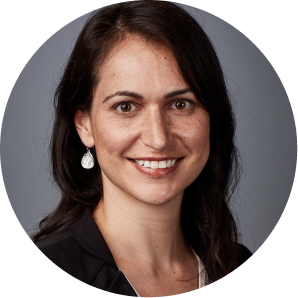
Liz Berman is an Expert on the Catalant platform with nearly 20 years of experience in Marketing, Strategy, Customer Insights, and Analytics. Liz has worked for CPG and Retail leaders such as PepsiCo, Safeway/Albertsons, Peet’s Coffee, and Rodan + Fields and is now an independent consultant. She teaches a course at Stanford Continuing Studies on Customer Loyalty & Retention. Liz has an MBA from Harvard Business School and a BA in Economics summa cum laude from Yale University.
Want to work directly with Liz Berman? Contact us to learn how.
Find CPG and retail marketing Experts now
Welcome to the Era Catalant Calls Consulting 2.0
It’s a modern, digitally-enabled approach to solving complex business problems, giving leaders (even those outside of the C-suite) direct access through a technology platform to the expertise of business professionals, like Liz Berman, who have the skills required to drop in on-demand and execute strategic work. It’s no longer sufficient to throw smart, but inexperienced, people onto a complex problem. Digital connectedness, combined with the rapid migration of top talent into freelance consulting, allows business leaders to find someone who’s already solved their exact problems before.